eBook
AFP Executive Guide to Identifying Value for Treasury Automation, Machine Learning & Artificial Intelligence
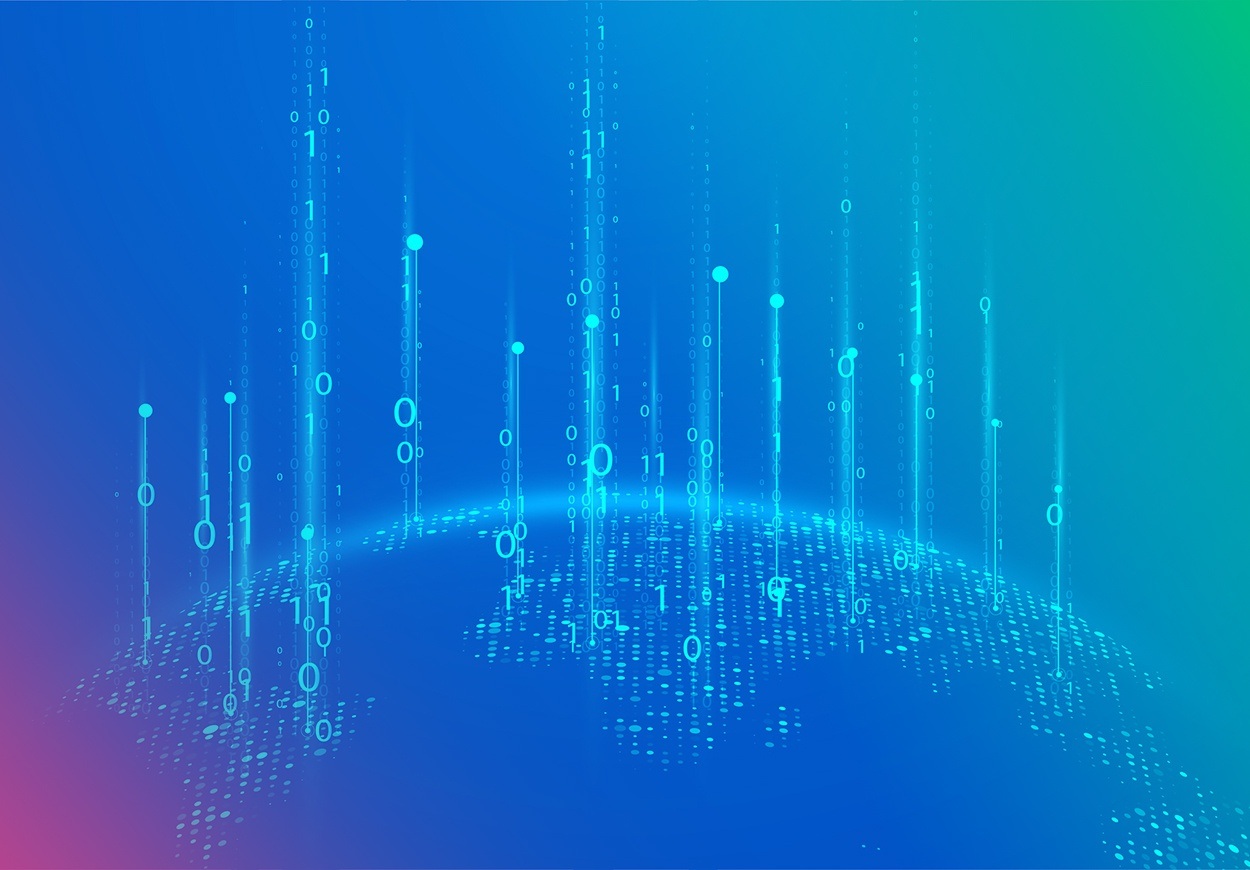
Digital treasury tools, such as robotic process automation (RPA), machine learning and artificial intelligence (AI) are already being used to facilitate treasury automation. The use of treasury technology leads to better decision-making and also frees time for skilled treasury practitioners to focus on strategic development.
This guide outlines how RPA, ML and AI can–and are–being used to improve treasury management processes for receivables finance, payments, fraud detection and more. The guide also explores how to build a business case for a new automation project.
Table of Contents
The Need for Technology to Maintain Effective Operations
Corporate treasury departments rely on technology to maintain effective operations. The technology varies and is constantly improving, offering ever more sophisticated solutions and functionality.
Treasury uses a wide range of different technologies, from spreadsheets developed in-house to manage a specific process to highly sophisticated treasury management systems. Technology is also an enabler as treasury evolves from an operational department to a strategic partner to the whole of the business, with automation playing an increasingly important role.
While technology is critical to improving operational efficiency, that efficiency is only achievable if the technology is deployed to perform suitable tasks, which requires accurate expectations of what each type of technology can and, just as importantly, cannot deliver. Treasurers must be able to do two things:
- Identify key inefficiencies, or “pain” points, within their operations.
- Match each activity to an appropriate technology with the potential to solve them.
Pain points manifest themselves in different ways, whether as errors, such as missed investment opportunities or unhedged exposures, or as timeconsuming manual processes, such as the preparation of the cash position. While the root causes and associated operational weakness are relatively simple to identify, the challenge lies in selecting appropriate solutions to solve those problems.
All companies have these pain points and, if left unchecked, they are only going to get worse. With the increased use of real-time payments, we are moving ever nearer to a world of real-time finance, an environment of constantly changing data. Without more automation, treasury practitioners simply will not be able to make decisions quickly enough.
On a positive note, digital treasury tools, notably Robotic Process Automation (RPA) and machine learning, are already being used to facilitate treasury automation. The use of these tools can be shown to lead to better decision-making; it also frees time for skilled treasury practitioners to focus on strategic development. This guide outlines how RPA and machine learning can, and is, being used, and shows how to build a business case for a new automation project.
Robotic Process Automation, Machine Learning and Artificial Intelligence
In this section, RPA and machine learning/artificial intelligence (AI) are defined and explained along with the potential benefits of their use. The next section includes three use cases to highlight how different companies have already deployed digital technologies to streamline operations.
Robotic Process Automation
Robotic Process Automation is a rules-based technology enabling users to automate repetitive tasks. It effectively uses a software “bot” to replicate a series of manual processes performed by a person. Unlike a standard workflow process that operates within a single system, an RPA bot can be set up to capture data from multiple systems, as it mirrors a human treasury team member by sitting above existing systems. Because of this, RPA processes can often be implemented quickly and without major disruption to existing operations. RPA is typically used to replicate a manual, repetitive task, or series of tasks, that can be tightly defined.
Machine Learning and Artificial Intelligence
Artificial intelligence (AI) is the use of a computer or machine to mimic certain elements of human intelligence. Machine learning (ML) is a branch of AI, in which a machine learns how to identify patterns in data.
Unlike RPA, which simply replicates a series of repetitive processes, machine learning can be used to analyze data to identify trends or patterns, via the use of algorithms. This goes to the heart of many companies’ problems with data analysis: companies generally hold, or amass vast stores of data, but they do not convert it into meaningful information.
As with RPA, data analysis via machine learning is faster than human computation; once set up, machine learning can operate any time (constantly, overnight or according to a customized schedule), enabling decisions to be made with the optimal, latest available data.
However, implementation is more complex than typical RPA scenarios. Machines can learn, or be taught via amendments to the underlying algorithms, patterns over time, but they are reliant on access to multiple data to perform meaningful calculations. Companies need to be prepared to make the investment in technology and data cleansing or preparation before any machine learning would be effective, both from a results and a cost perspective.
The Potential Benefits of RPA and Machine Learning
Although the use cases vary, the use of RPA and machine learning offer similar potential advantages, including:
- Improved accuracy. With RPA, as long as the process is set up correctly, the bot will perform the same tasks in the same way every time. The risk of human processing errors is eliminated and, if any variance between RPA outcome and actual outcome is identified, the RPA process can be adjusted. In the case of machine learning, accuracy will improve over time, as the machine learns and the algorithms are adjusted.
- Significantly reduced processing time. Bots and machines can perform typical tasks in a fraction of the time it takes a person to complete. This means that activities can be performed faster, so decisions can be made on the most recent available data.
- Results available, globally, when needed. Machine learning and RPA technology can operate at any time, so calculations can be performed overnight or on desired schedules to meet operational requirements. So, for example, in the case of cash positioning in a multinational organization, results can be available when teams in each location start their respective days, rather than two or more hours into them.
- Time management. Eliminating mundane processing from a treasury professional’s day frees that time to devote to more value-added activities, whether that is engaging with additional timesaving activities, supporting the wider business or focusing on strategic decisions.
- Improved morale. Although some treasury staff will be concerned about the impact of RPA and machine learning on their own jobs, for most organizations the technology will be an additional process that will improve team members’ experiences by eliminating the stress of calculating positions under time pressure or reducing the risk of error. Team members will also have time to spend on more interesting and personally rewarding activities.
How Emerging Technologies Are Being Used
This section outlines three ways RPA and machine learning are being used to solve particular problems faced by individual treasury departments.
Case Study: Automating Time-Consuming Tasks via RPA
One insurance company’s treasury department used to spend hundreds of hours a year processing internal customer requests for check images. While critical for the business as a whole, these requests were timeconsuming to complete and added no value within finance.
The treasury team developed an internal RPA process via bot to automate the process. While the team had to spend some time training their internal customers on the new process so that requests were made in a standardized format to enable bot processing, the system is now operational and running three times a day.
Using the bot has improved the response time for treasury’s internal customers, while releasing time back to treasury to devote to more value-added activities. It is now a task that treasury no longer has to perform. In addition, the customer experience and SLA have improved, as treasury can now typically respond to a customer’s request in a matter of hours, rather than weeks.
Case Study: Improving Cash Flow Forecasts
One of the key benefits of RPA is that it can be used to process data from a number of different company systems. This makes RPA a useful tool to improve cash flow forecasts, as they are built on data sourced from banks, treasury management and ERP systems and from other company departments, including payment teams.
Séverine Le Blevennec, senior director of EMEA Treasury at Honeywell has led the development of an RPA process to improve the accuracy and timeliness of the in-house bank’s cash flow forecasts. She identified RPA as a technology that might have the capability to enhance the existing forecast, and then took time to fully understand whether RPA could work by examining the technology in some detail.
Convinced that RPA was the potential solution, Le Blevennec then engaged with internal and external stakeholders to communicate her vision. She worked with Honeywell’s technology providers and banking partners to see whether data could be supplied in a more accessible way, wanting her colleagues to view the bot as a useful colleague, not as a threat to their jobs.
Critically, Le Blevennec knew the bot needed to have a significant positive impact from the start, as she wanted the project to energize her colleagues toward future digitization projects. In other words, the new process had to deliver the expected returns.
Ensuring this required a review of the current process and a strict testing program. Le Blevennec learned that “rule-based programming [like RPA] requires detailed documentation.” She revisited all existing processes and created seven workflows for seven different activities, from maturing time deposits to intraday payments and collections. She emphasized the importance of testing. Tests were performed in a mindset in which it was expected that “things could go wrong.” Then, before going live, the team used the new spreadsheet alongside the old process to make sure everything worked as expected.
Since going live, Honeywell has seen some significant benefits. The new system is more efficient: Two hours a day have been saved. It is more accurate: The old manual system could only include data from about 40 Honeywell bank accounts. Today, data from over 160 accounts are included in the forecast, and any new accounts can be included easily. Enhanced visibility has resulted in improved counterparty risk management, reduced levels of un-invested cash and, as a result, increased investment returns.
Most importantly, Honeywell’s treasury team has seen the benefits. They are less stressed, and more engaged for the next stage in Honeywell’s digitization journey.
Case Study: Receivables Management
A steady growth in sales, resulting in increased receivables, is usually good news. But it might not be for an AR team, over-reliant on expensive lockbox processing. With over 2,500 monthly checks all needing some form of human intervention coupled with increased sales, a technology company’s AR team suffered from low morale, leading to delays in receivables processing and reduced confidence in the accuracy of AR data in the ERP. As a result, the treasury team was so busy processing payments, they didn’t have time to convince customers to transition to more efficient, less costly electronic payment formats.
The team recognized they needed a solution that could be scaled and that would allow them to react in the ever-changing B2B payment environment. It is one thing to recognize the need for change; it is another to understand how to bring it about. The team did their research and spoke to their banking partners. One discussion started with an enquiry on how to implement a more modern lockbox, utilizing the OCR codes; it ended with the team realizing that machine learning could be used to automate some of the processes.
They were able to design a solution that would get machines to do much of the previously manual work. However, there were hurdles to overcome. For example, in one division, clients tended to pay by claim, rather than by invoice, and the ERP system didn’t hold the claim line item information. The team realized bridging the gap between the system holding the claim information and the ERP was well-suited to machine learning.
Once the solution was operational, it freed time for the team to manage exceptions and also to improve the quality of the data on which the AI system relied. The time savings enabled the team to work with customers to send and enhance information coming into the system, so the machine learning tool could better consolidate and match the data, and constantly learn to improve.
The result was dramatic. By replacing the manual gathering, consolidation and formatting that was required every morning, the AI-enabled receivables solution allows the company to quickly improve the time taken to process a payment. Most payments are processed within two days. This was achieved because of the consolidation of information. The payments are now standardized. Reconciliation is simpler, with the team confident all the information is there.
Three months later, with even more time available as the machine continues to learn from manual exception management actions, the team can spend more time with collections and customers to help them provide better remittance information, further improving matching. The team can now build electronic adoption, with the AI bringing together remittance and payment automatically. Now, every time a match is confirmed, the system can see it and learn from it.
The team can respond quickly to queries. Information is tracked immediately, so there is no need for time-consuming searches for data. The team has confidence in the data, and morale is high as they can focus on more value-added activities.
Making the Business Case to Implement
As with any technology project, it is critical to build a strong business case when seeking to adopt RPA or machine learning. This means getting buy-in from a project sponsor, and approval from all required stakeholders. Setting and achieving key success metrics will give credibility to the project, which in turn will help treasury practitioners introduce more digital finance initiatives. To help make a compelling business case and ensure the objectives are well defined, there are a number of key considerations.
Understand the technology to maximize the potential benefits. As outlined above, different technologies are better suited to solving particular problems. The project owner needs to understand the nature of the problem and how the proposed technology will solve it. Some proposals may appear to be a standalone solution to a particular problem with limited impact across the wider business, yet when examined further, are either extendible into other functions and/or require change to operations within those functions.
Optimize processes before automating. If there is an existing process, map it and review whether it can be made more efficient. Many manual processes incorporate separate checks and approvals to protect against error and fraud. While some may need to be migrated into an automated process, for example if a transaction exceeds a certain pre-set limit, it may not be necessary to migrate all of them, as long as the rules are tightly written. A machine learning project may require improvements to data management to enable automated data analysis.
Communicate and educate stakeholders on the proposed solution. Communication is central to the success of any project. Senior management will have to approve the project. If IT input is required, they will need to be engaged early in the planning process to secure resources. Banks, technology providers and other data suppliers should also be approached early to plan how they can support the project. Treasury team members will want to understand the implications for them.
Identify potential returns. One of the key benefits of automating a process, whether by RPA or ML, is to remove layers of human involvement in either mundane, standardized processing or time-consuming data collation and analysis. So, although there will be some clearly measurable costs and benefits, many will be “softer” benefits in the form of released time and reduced risk of error and fraud.
Establish and monitor success metrics. It can be helpful to identify some clear targets to serve as measurements of the success of the project, such as how much time an RPA project has saved. It may be possible to illustrate consequential benefits too, such as improved investment returns due to more accurate cash forecasts. If possible, use these measurements to refine the bot or machine to achieve further efficiencies.
Scale the solution; look for the next step. Measuring the outcome of one project will help build support and momentum for others. As technology develops, there will always be further ways it can be adopted to improve treasury operations.
Greater Automation Is the Future
As this guide has indicated, technology is enabling corporate treasury departments to operate more efficiently by automating processes and taking advantage of more advanced data analytics. In turn, these changes further enable transformation and evolution of treasury departments from laborintensive operational, tactical departments into strategic partners to the wider business.
The adoption of new treasury technologies continues to accelerate, driven by multiple factors. Two stand out: the evolution of the “internet of things,” and the move toward “real-time” finance. While the catalysts for the development of these two trends is different, the implications for treasury and finance are closely linked.
The Internet of Things
The value of the internet of things comes from the way data can be shared between billions of different devices being connected via the internet. It allows individuals to control their personal environment (e.g., smart lighting and heating) and companies to manage a whole range of processes from stock ordering to logistics management. For treasury and finance, the value will come from being able to link the physical and financial supply chains and gain better insight into cash. To do so effectively, data sent out by these connected devices needs to be analyzed by artificial intelligence; the devices simply produce too much data to be analyzed in any other way.
The Implications of Real-Time Finance
There is a clear trend toward more real-time activity in treasury and finance, with real-time payments being just one, albeit significant, step. Notably, the move toward real-time processing is also a shift to 24/7/365, always on operations. Treasury will need to consider how to manage this change and, particularly, how to manage risks that will emerge overnight, including between the Friday close and the Monday restart.
The growth of e-commerce has already provided a sense of changes to come. Consumers who pay online expect to see their order status updated in real time and, in some sectors, the service or product available in real time too. With the adoption of real-time payments, actions not limited to fraud prevention have to take place in real time as part of the payment initiation process, as real-time payments are generally irrevocable, with no opportunity to stop or amend payments.
But managing payment processes is just one part of a much wider change that the adoption of realtime payments will bring to finance and treasury. If payments are being made in real time, treasury departments will need to manage their liquidity in real time too and they will rely on a level of automation and artificial intelligence to do so. And before long, the foreign exchange and money markets will move toward real time, profoundly affecting the treasury departmental day, which is currently structured by cut-off times.
For these reasons, it seems inevitable that both RPA and artificial intelligence, including machine learning, will play a more prominent role in the management of corporate treasury departments in the coming years.
Conclusion
Although RPA and AI are seen as cutting edge, in reality many companies are benefiting from these technologies through solutions provided by their banks and technology partners. For organizations yet to implement the technology, doing so successfully requires three key steps:
- Identify a pain point to be solved. RPA and AI/machine learning both work best when implemented to solve particular problems. Don’t start using RPA; start using RPA to automate a process.
- Match the technology to the task. Different types of technology solve different problems. If you want to automate a process, RPA is likely to be the more suitable solution. If you want to analyze data, investigate AI, including machine learning.
- Build a business case. RPA and AI/ ML are likely to become even more important in the future, so a successful first project is important. Make sure your chosen technology can do what you want it to do, then build support for your solution among stakeholders including, critically, the treasury team. You can then use your successful first project as the springboard for future development.