Blog
AI in Liquidity Management: How It Can Assist a Liquidity Manager
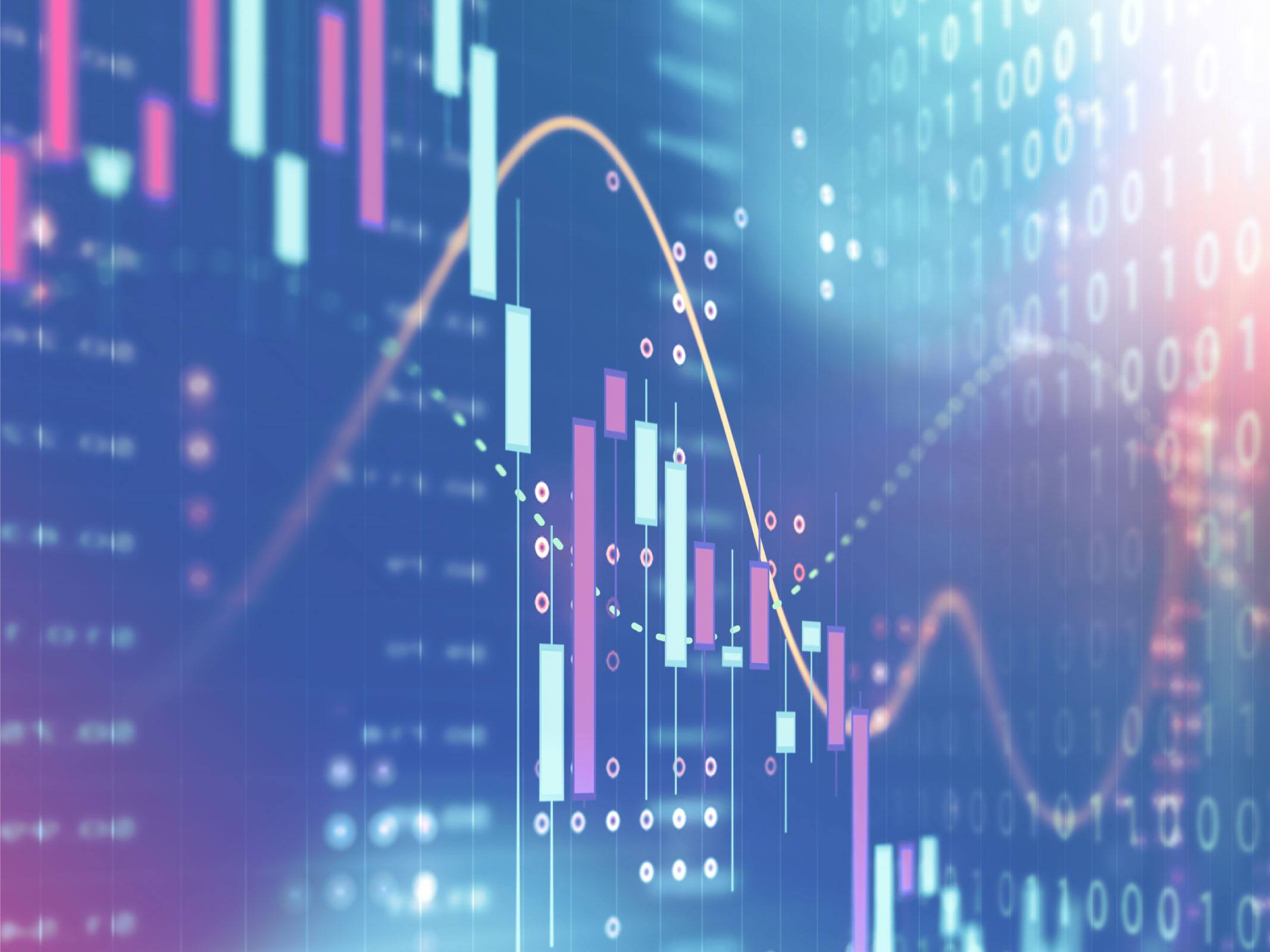
Artificial intelligence and machine learning (AI/ML) are emerging treasury technology that have become essential for cash flow forecasting, payments fraud prevention and liquidity management. But is treasury on board and ready for AI in liquidity management?
The Difference between ML and AI
AI and ML are not technically the same thing. AI is a broad term denoting intelligent machines that can augment human capabilities. ML is an application or subset of AI that enables machines to learn from data without additional programming.
Perhaps the most important difference is that AI provides prescriptive analytics that help us choose the next best action. ML models, in contrast, provide predictive analytics by being trained on historical data. The system then runs new data through the trained ML model to make much more informed predictions.
ML models are much more powerful than they were just a few years ago due to the amount of data treasury and finance teams have at their disposal. Companies and consumers use a host of applications that generate mass amounts of data. With the right systems in place, organizations can use that data for better decision-making.
“The larger your set of historical data is, the better the trained machine learning model will be,” said Jean-Baptiste Gaudemet, SVP of data and analytics for Kyriba, during a recent webinar.
Why Use ML and AI in Treasury Management
AI/ML provides treasury and finance with insights into trends and activities that were previously challenging to set up and see. In particular, AI capabilities can help shape cash reporting. For example:
- Foreign currency balances in the APAC region continued to increase in Q1. What can we expect for Q2?
- Cash receipts volume for one month jumped dramatically for a specific customer group. How does that compare to the prior six months?
- Surplus cash always drops during the months of July and August for specific accounts or regions/countries. Why these months and these accounts?
- Payment flows have shifted unexpectedly in Q3, and we have not seen this level of payment for Q3 for the past three years. What has caused this dramatic shift?
- The company has a cash surplus. What is the risk of overdraft if that surplus is used for a dynamic discounting program?
Organizations can increase the accuracy of their short-term forecasts with AI-based tools that learn from the history of cash flows and continuously improve inflow projections over time. With deeper analysis of this data, organizations can better predict cash flows by season or region, which in turn reduces efforts for key functions like accounts payable by, anticipating free cash flow closing, and adjusting the payment campaign budget.
Gaudemet noted that manual processes persist in treasury, even with the advent and evolution of technologies like AI/ML. Many treasury departments continue to rely on Excel, even though it can produce highly inaccurate cash flow forecasts that can negatively impact the business. “Despite the development and maturity of these technologies, we still see a lot of treasurers stuck with Excel,” he said. “Spreadsheets are basically manual input, which means there are very frequently a lot of errors. They’re highly person dependent, and it is impossible to implement an ML algorithm in Excel.”
AI/ML users also can select what companies, currencies and cash flow types to include, as well as adjust the forecasting period to align on short-term payment/funding/investment decisions. With interest rates increasing, treasury teams can optimize liquidity by reducing their maximum idle cash while also minimizing the risk and cost of overdraft. Treasury will be able to determine how much of its budget it can allocate towards certain expenditures over a period of time, or whether it will need to borrow funds to make certain payments.
Kyriba has implemented this technology to allow clients to make more informed decisions. The AI/ML engine is trained on data and makes this meaningful information available not only for cash reporting, but for all applications to include liquidity forecasting, financial risk management, financial transactions, payments fraud prevention, payment optimization, bank relationship management and more.
How Machine Learning Works for Treasury
“Kyriba is the largest network for liquidity data,” Gaudemet said. “We connect to over 1,000 banks. We have connectivity with most ERPs, which, when combined together gives our customers access to working capital, transactional ERP information and cash and liquidity to get a more complete Enterprise Liquidity view.“ Kyriba’s connectivity with financial platforms, which integrates with, and collects transactional trading data, completes the liquidity picture and delivers the information to the data warehouse, which is then consumed in real-time on the data analytics platform to produce actionable analytics.”
The Future of AI in Liquidity Management
With more data than ever at our disposal, now is the time for treasury and finance departments to embrace AI/ML. Moving on from manual processes not only makes treasury’s job much easier—it increases accuracy, benefiting the entire organization. Companies that want to survive and thrive in today’s rapidly changing environment need the best tools available.
Learn More:
- Bitesize Webinar: The Role of AI in Liquidity Management
- eBook: Artificial Intelligence: Creating Treasury’s Future, Today
- Fact Sheet: Kyriba Cash Management AI